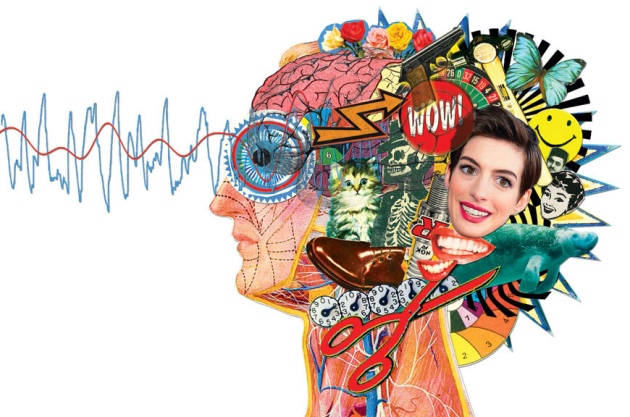
From Nature News, Kerri Smith offers an overview of the current research by neuroscientists who are seeking to decode human thoughts, dreams, and intentions. The trick is to convert neuronal electrical patterns of synapses, networks, and modules into something that appears coherent to us.
Full Citation:
Smith, K. (2013, Oct 24). Brain decoding: Reading minds. Nature, 502(7472): 428–430. doi:10.1038/502428a
Brain decoding: Reading minds
By scanning blobs of brain activity, scientists may be able to decode people's thoughts, their dreams and even their intentions.
Kerri Smith
23 October 2013
Cracking the code - See how scientists decode vision, dreamscapes and hidden mental states from brain activity.
Jack Gallant perches on the edge of a swivel chair in his lab at the University of California, Berkeley, fixated on the screen of a computer that is trying to decode someone's thoughts.
On the left-hand side of the screen is a reel of film clips that Gallant showed to a study participant during a brain scan. And on the right side of the screen, the computer program uses only the details of that scan to guess what the participant was watching at the time.
Anne Hathaway's face appears in a clip from the film Bride Wars, engaged in heated conversation with Kate Hudson. The algorithm confidently labels them with the words 'woman' and 'talk', in large type. Another clip appears — an underwater scene from a wildlife documentary. The program struggles, and eventually offers 'whale' and 'swim' in a small, tentative font.
“This is a manatee, but it doesn't know what that is,” says Gallant, talking about the program as one might a recalcitrant student. They had trained the program, he explains, by showing it patterns of brain activity elicited by a range of images and film clips. His program had encountered large aquatic mammals before, but never a manatee.
Groups around the world are using techniques like these to try to decode brain scans and decipher what people are seeing, hearing and feeling, as well as what they remember or even dream about.
Listen
Neuroscientists can predict what a person is seeing or dreaming by looking at their brain activity.
Go to full podcastMedia reports have suggested that such techniques bring mind-reading “from the realms of fantasy to fact”, and “could influence the way we do just about everything”. The Economist in London even cautioned its readers to “be afraid”, and speculated on how long it will be until scientists promise telepathy through brain scans. Although companies are starting to pursue brain decoding for a few applications, such as market research and lie detection, scientists are far more interested in using this process to learn about the brain itself. Gallant's group and others are trying to find out what underlies those different brain patterns and want to work out the codes and algorithms the brain uses to make sense of the world around it. They hope that these techniques can tell them about the basic principles governing brain organization and how it encodes memories, behaviour and emotion (see 'Decoding for dummies').
Applying their techniques beyond the encoding of pictures and movies will require a vast leap in complexity. “I don't do vision because it's the most interesting part of the brain,” says Gallant. “I do it because it's the easiest part of the brain. It's the part of the brain I have a hope of solving before I'm dead.” But in theory, he says, “you can do basically anything with this”.
Beyond blobology
Brain decoding took off about a decade ago1, when neuroscientists realized that there was a lot of untapped information in the brain scans they were producing using functional magnetic resonance imaging (fMRI). That technique measures brain activity by identifying areas that are being fed oxygenated blood, which light up as coloured blobs in the scans. To analyse activity patterns, the brain is segmented into little boxes called voxels — the three-dimensional equivalent of pixels — and researchers typically look to see which voxels respond most strongly to a stimulus, such as seeing a face. By discarding data from the voxels that respond weakly, they conclude which areas are processing faces.
Decoding techniques interrogate more of the information in the brain scan. Rather than asking which brain regions respond most strongly to faces, they use both strong and weak responses to identify more subtle patterns of activity. Early studies of this sort proved, for example, that objects are encoded not just by one small very active area, but by a much more distributed array.
These recordings are fed into a 'pattern classifier', a computer algorithm that learns the patterns associated with each picture or concept. Once the program has seen enough samples, it can start to deduce what the person is looking at or thinking about. This goes beyond mapping blobs in the brain. Further attention to these patterns can take researchers from asking simple 'where in the brain' questions to testing hypotheses about the nature of psychological processes — asking questions about the strength and distribution of memories, for example, that have been wrangled over for years. Russell Poldrack, an fMRI specialist at the University of Texas at Austin, says that decoding allows researchers to test existing theories from psychology that predict how people's brains perform tasks. “There are lots of ways that go beyond blobology,” he says.
In early studies1, 2 scientists were able to show that they could get enough information from these patterns to tell what category of object someone was looking at — scissors, bottles and shoes, for example. “We were quite surprised it worked as well as it did,” says Jim Haxby at Dartmouth College in New Hampshire, who led the first decoding study in 2001.
Soon after, two other teams independently used it to confirm fundamental principles of human brain organization. It was known from studies using electrodes implanted into monkey and cat brains that many visual areas react strongly to the orientation of edges, combining them to build pictures of the world. In the human brain, these edge-loving regions are too small to be seen with conventional fMRI techniques. But by applying decoding methods to fMRI data, John-Dylan Haynes and Geraint Rees, both at the time at University College London, and Yukiyasu Kamitani at ATR Computational Neuroscience Laboratories, in Kyoto, Japan, with Frank Tong, now at Vanderbilt University in Nashville, Tennessee, demonstrated in 2005 that pictures of edges also triggered very specific patterns of activity in humans3, 4. The researchers showed volunteers lines in various orientations — and the different voxel mosaics told the team which orientation the person was looking at.
ILLUSTRATION BY PETER QUINNELL; PHOTO: KEVORK DJANSEZIAN/GETTY
Edges became complex pictures in 2008, when Gallant's team developed a decoder that could identify which of 120 pictures a subject was viewing — a much bigger challenge than inferring what general category an image belongs to, or deciphering edges. They then went a step further, developing a decoder that could produce primitive-looking movies of what the participant was viewing based on brain activity5.
From around 2006, researchers have been developing decoders for various tasks: for visual imagery, in which participants imagine a scene; for working memory, where they hold a fact or figure in mind; and for intention, often tested as the decision whether to add or subtract two numbers. The last is a harder problem than decoding the visual system says Haynes, now at the Bernstein Centre for Computational Neuroscience in Berlin, “There are so many different intentions — how do we categorize them?” Pictures can be grouped by colour or content, but the rules that govern intentions are not as easy to establish.
Gallant's lab has preliminary indications of just how difficult it will be. Using a first-person, combat-themed video game called Counterstrike, the researchers tried to see if they could decode an intention to go left or right, chase an enemy or fire a gun. They could just about decode an intention to move around; but everything else in the fMRI data was swamped by the signal from participants' emotions when they were being fired at or killed in the game. These signals — especially death, says Gallant — overrode any fine-grained information about intention.
The same is true for dreams. Kamitani and his team published their attempts at dream decoding in Science earlier this year6. They let participants fall asleep in the scanner and then woke them periodically, asking them to recall what they had seen. The team tried first to reconstruct the actual visual information in dreams, but eventually resorted to word categories. Their program was able to predict with 60% accuracy what categories of objects, such as cars, text, men or women, featured in people's dreams.
The subjective nature of dreaming makes it a challenge to extract further information, says Kamitani. “When I think of my dream contents, I have the feeling I'm seeing something,” he says. But dreams may engage more than just the brain's visual realm, and involve areas for which it's harder to build reliable models.
Reverse engineering
Decoding relies on the fact that correlations can be established between brain activity and the outside world. And simply identifying these correlations is sufficient if all you want to do, for example, is use a signal from the brain to command a robotic hand (see Nature 497, 176–178; 2013). But Gallant and others want to do more; they want to work back to find out how the brain organizes and stores information in the first place — to crack the complex codes the brain uses.
That won't be easy, says Gallant. Each brain area takes information from a network of others and combines it, possibly changing the way it is represented. Neuroscientists must work out post hoc what kind of transformations take place at which points. Unlike other engineering projects, the brain was not put together using principles that necessarily make sense to human minds and mathematical models. “We're not designing the brain — the brain is given to us and we have to figure out how it works,” says Gallant. “We don't really have any math for modelling these kinds of systems.” Even if there were enough data available about the contents of each brain area, there probably would not be a ready set of equations to describe them, their relationships, and the ways they change over time.
Computational neuroscientist Nikolaus Kriegeskorte at the MRC Cognition and Brain Sciences Unit in Cambridge, UK, says that even understanding how visual information is encoded is tricky — despite the visual system being the best-understood part of the brain (see Nature 502, 156–158; 2013). “Vision is one of the hard problems of artificial intelligence. We thought it would be easier than playing chess or proving theorems,” he says. But there's a lot to get to grips with: how bunches of neurons represent something like a face; how that information moves between areas in the visual system; and how the neural code representing a face changes as it does so. Building a model from the bottom up, neuron by neuron, is too complicated — “there's not enough resources or time to do it this way”, says Kriegeskorte. So his team is comparing existing models of vision to brain data, to see what fits best.
Real world
Devising a decoding model that can generalize across brains, and even for the same brain across time, is a complex problem. Decoders are generally built on individual brains, unless they're computing something relatively simple such as a binary choice — whether someone was looking at picture A or B. But several groups are now working on building one-size-fits-all models. “Everyone's brain is a little bit different,” says Haxby, who is leading one such effort. At the moment, he says, “you just can't line up these patterns of activity well enough”.
Standardization is likely to be necessary for many of the talked-about applications of brain decoding — those that would involve reading someone's hidden or unconscious thoughts. And although such applications are not yet possible, companies are taking notice. Haynes says that he was recently approached by a representative from the car company Daimler asking whether one could decode hidden consumer preferences of test subjects for market research. In principle it could work, he says, but the current methods cannot work out which of, say, 30 different products someone likes best. Marketers, he says, should stick to what they know for now. “I'm pretty sure that with traditional market research techniques you're going to be much better off.”
Companies looking to serve law enforcement have also taken notice. No Lie MRI in San Diego, California, for example, is using techniques related to decoding to claim that it can use a brain scan to distinguish a lie from a truth. Law scholar Hank Greely at Stanford University in California, has written in the Oxford Handbook of Neuroethics (Oxford University Press, 2011) that the legal system could benefit from better ways of detecting lies, checking the reliability of memories, or even revealing the biases of jurors and judges. Some ethicists have argued that privacy laws should protect a person's inner thoughts and desires as private, but Julian Savulescu, a neuroethicist at the University of Oxford, UK, sees no problem in principle with deploying decoding technologies. “People have a fear of it, but if it's used in the right way it's enormously liberating.” Brain data, he says, are no different from other types of evidence. “I don't see why we should privilege people's thoughts over their words,” he says.
Haynes has been working on a study in which participants tour several virtual-reality houses, and then have their brains scanned while they tour another selection. Preliminary results suggest that the team can identify which houses their subjects had been to before. The implication is that such a technique might reveal whether a suspect had visited the scene of a crime before. The results are not yet published, and Haynes is quick to point out the limitations to using such a technique in law enforcement. What if a person has been in the building, but doesn't remember? Or what if they visited a week before the crime took place? Suspects may even be able to fool the scanner. “You don't know how people react with countermeasures,” he says.
Other scientists also dismiss the implication that buried memories could be reliably uncovered through decoding. Apart from anything else, you need a 15-tonne, US$3-million fMRI machine and a person willing to lie very still inside it and actively think secret thoughts. Even then, says Gallant, “just because the information is in someone's head doesn't mean it's accurate”. Right now, psychologists have more reliable, cheaper ways of getting at people's thoughts. “At the moment, the best way to find out what someone is going to do,” says Haynes, “is to ask them.”
References
1. Haxby, J. V. et al. Science 293, 2425–2430 (2001). Article
2. Cox, D. D. & Savoy, R. L. et al. NeuroImage 19, 261–270 (2003). Article
3. Haynes, J.-D. & Rees, G. Nature Neurosci. 8, 686–691 (2005). Article
4. Kamitani, Y. & Tong, F. Nature Neurosci. 8, 679–685 (2005). Article
5. Nishimoto, S. et al. Curr. Biol. 21, 1641–1646 (2011). Article
6. Horikawa, T., Tamaki, M., Miyawaki, Y. & Kamitani, Y. Science 340, 639–642 (2013). Article
Related stories and links from Nature.com
- Scientists read dreams - 19 October 2012
- Neuroscience: The mind reader - 13 June 2012
- Brain imaging: fMRI 2.0 - 04 April 2012
- Mind-reading with a brain scan - 05 March 2008
- I know what you're thinking... - 08 February 2007
- Neuropod: Neuroscience podcasts from Nature
No comments:
Post a Comment